

We demonstrate the effectiveness of our new approach via experiments based on real-world data and simulations. To improve the learning efficiency of HAD-GNN, we also extend the mini-batch gradient descent to inductive representation learning on such dynamic graphs and adopt a t-batch training mechanism (t-BTM). We develop hybrid-attention dynamic graph neural network (HAD-GNN), an inductive graph representation learning framework for dynamic stock graphs to incorporate temporal and graph attention mechanisms. This paper represents the first effort to leverage such phenomena to construct dynamic stock graphs for stock predictions. As a result, firms’ stock prices often feature synchronous co-movement patterns.

In today’s economy, individual firms are increasingly connected via different types of relationships. Summary of Contribution: Accurate predictions of stock prices have important implications for financial decisions. The proposed method can have important implications for the management of financial portfolios and investment risk. Extensive experiments on stocks from different markets and trading simulations demonstrate that the proposed method significantly improves stock predictions.
GOOGLE SHEETS NEWSFLOW UPDATE
We also extended mini-batch gradient descent to inductive representation learning on dynamic stock graphs so that the model can update parameters over mini-batch stock graphs with higher training efficiency. To learn node representations from such dynamic graphs for better stock predictions, we propose the hybrid-attention dynamic graph neural network, an inductive graph representation learning method. This paper proposes a novel method to leverage such relationships for stock price predictions by adopting inductive graph representation learning on dynamic stock graphs constructed based on historical stock price co-movement. Overall, these findings suggest that biased public information inherent in news sentiment serves to irrationalize investors' acquisition of firm-specific information through a biased perception of uncertainties in the risky asset payoff.Ĭo-movement among individual firms’ stock prices can reflect complex interfirm relationships. Second, the return predictability from firm-specific news sentiment confirms that it contributes to variations in asset information risk due, in a biased belief equilibrium, to the proportion of informed investors deviating from rational expectations. First, when sentiment in news articles, as a proxy for biased public information, is more optimistic, investors tend to acquire less earnings-relevant information before the earnings announcement and vice versa. This model of information acquisition yields testable predictions that are verified by using a novel dataset of news stories. Investors who use biased information from news media subsequently tend to make irrational decisions about acquiring firm-specific information compared to rational expectations. The results point towards strong divergence of expectations between US and Asian real estate companies in the onset of Covid-19, which may be associated with the level of prior experience to similar pandemics. Firms in the US perform significantly worse if their exposure to the pandemic is higher, which is not the case in Asia. A Fama–MacBeth regression shows evidence for a low-risk effect during the Covid period: while insignificant prior to the pandemic, the return-risk relationship becomes significantly negative during the Covid period, with valuation effects driving the results in both regions. The most affected sectors in the US are retail and hotels, while in Asia it is office.
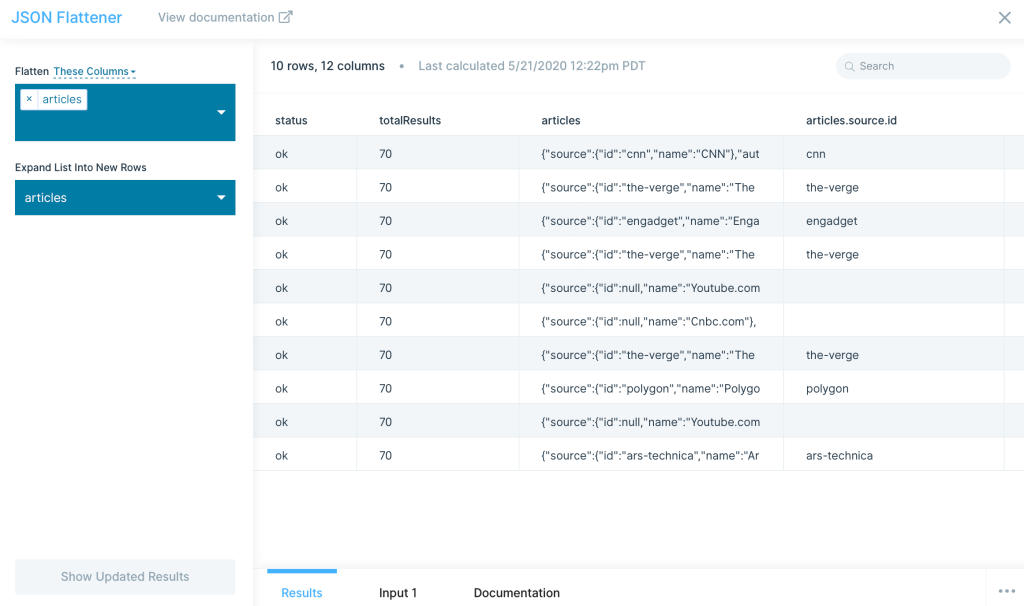
Real estate sectors experience strong divergence in performance in the US while little sectoral difference is observed in Asia. During the early stages of the pandemic, the sensitivity of Asian real estate companies to the market becomes negative, while it remains positive and increases in the US. I find substantial differences between stocks in Asia and the US as a result of the pandemic. I construct regional Covid-19 Risk Factors (CRFs) to assess how the risk exposure of stocks to the pandemic affects their performance.

This paper uses the global systemic shock associated with the outbreak of the novel coronavirus Covid-19 to assess the risk-return relationship in the cross-section of real estate equities in the US and in selected Asian countries.
